Machine Learning Will be one of the Best Ways to Identify Habitable Exoplanets
By Matt Williams
The field of extrasolar planet studies is undergoing a seismic shift. To date, 4,940 exoplanets have been confirmed in 3,711 planetary systems, with another 8,709 candidates awaiting confirmation. With so many planets available for study and improvements in telescope sensitivity and data analysis, the focus is transitioning from discovery to characterization. Instead of simply looking for more planets, astrobiologists will examine “potentially-habitable” worlds for potential “biosignatures.”
This refers to the chemical signatures associated with life and biological processes, one of the most important of which is water. As the only known solvent that life (as we know it) cannot exist, water is considered the divining rod for finding life. In a recent study, astrophysicists Dang Pham and Lisa Kaltenegger explain how future surveys (when combined with machine learning) could discern the presence of water, snow, and clouds on distant exoplanets.
Dang Pham is a graduate student with the David A. Dunlap Department of Astronomy & Astrophysics at the University of Toronto, where he specializes in planetary dynamics research. Lisa Kaltenegger is an Associate Professor in Astronomy at Cornell University, the Director of the Carl Sagan Institute, and a world-leading expert in modeling potentially habitable worlds and characterizing their atmospheres.

Water is something that all life on Earth depends on, hence its importance for exoplanet and astrobiological surveys. As Lisa Kaltenneger told Universe Today via email, this importance is reflected in NASA’s slogan – “just follow the water” – which also inspired the title of their paper:
“Liquid water on a planet’s surface is one of the smoking guns for potential life – I say potential here because we don’t know what else we need to get life started. But liquid water is a great start. So we used NASA’s slogan of “Just follow the water” and asked, how can we find water on the surface of rocky exoplanets in the Habitable Zone? Doing spectroscopy is time intensive, thus we are searching for a faster way to initially identify promising planets – those with liquid water on it.”
Currently, astronomers have been limited to looking for Lyman-alpha line absorption, which indicates the presence of hydrogen gas in an exoplanet’s atmosphere. This is a byproduct of atmospheric water vapor that’s been exposed to solar ultraviolet radiation, causing it to become chemically disassociated into hydrogen and molecular oxygen (O2) – the former of which is lost to space while the latter is retained.
This is about to change, thanks to next-generation telescopes like the James Webb (JWST), Nancy Grace Roman (RST), and Origins Space Telescope, as well as next-next-generation observatories like the Habitable Exoplanet Observatory (HabEx) and Large UV/Optical/IR Surveyor (LUVOIR). There are also ground-based telescopes that will become operational in the coming years, like the Extremely Large Telescope (ELT), the Giant Magellan Telescope (GMT), and the Thirty Meter Telescope (TMT).
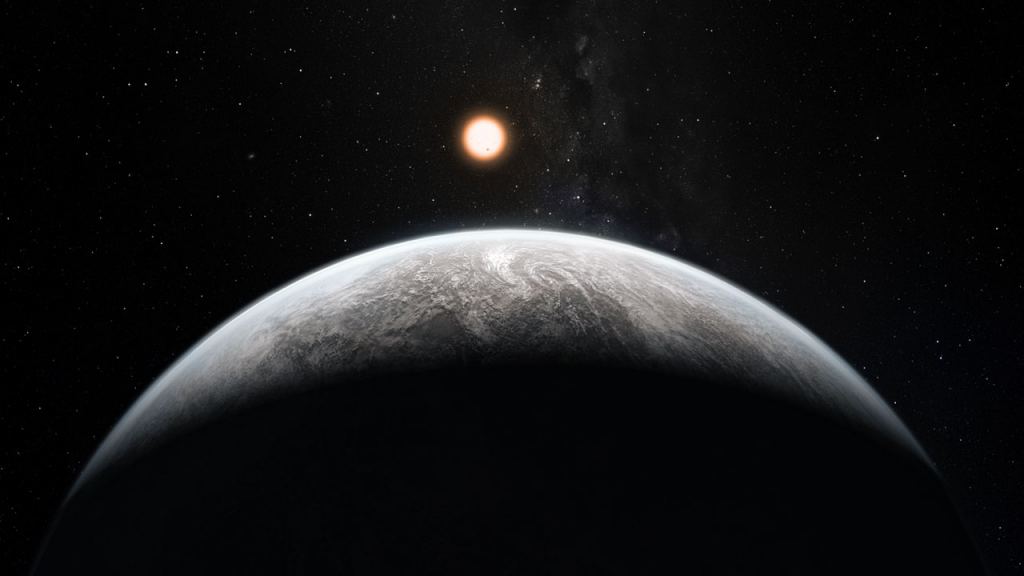
Thanks to their large primary mirrors and advanced suite of spectrographs, chronographs, adaptive optics, these instruments will be able to conduct Direct Imaging studies of exoplanets. This consists of studying light reflected directly from an exoplanet’s atmosphere or surface to obtain spectra, allowing astronomers to see what chemical elements are present. But as they indicate in their paper, this is a time-intensive process.
Astronomers start by observing thousands of stars for periodic dips in brightness, then analyzing the light curves for signs of chemical signatures. Currently, exoplanet researchers and astrobiologists rely on amateur astronomers and machine algorithms to sort through the volumes of data their telescopes obtain. Looking ahead, Pham and Kaltenneger show how more advanced machine learning will be crucial.
As they indicate, MI techniques will allow astronomers to conduct the initial characterizations of exoplanets more rapidly, allowing astronomers to prioritize targets for follow-up observations. By “following the water,” astronomers will be able to dedicate more of an observatory’s valuable survey time to exoplanets that are more likely to provide significant returns.
“Next-generation telescopes will look for water vapor in the atmosphere of planets and water on the surface of planets,” said Kaltenneger. “Of course, to find water on the surface of planets, you should look [for water in its] liquid, solid, and gaseous forms, as we did in our paper.”
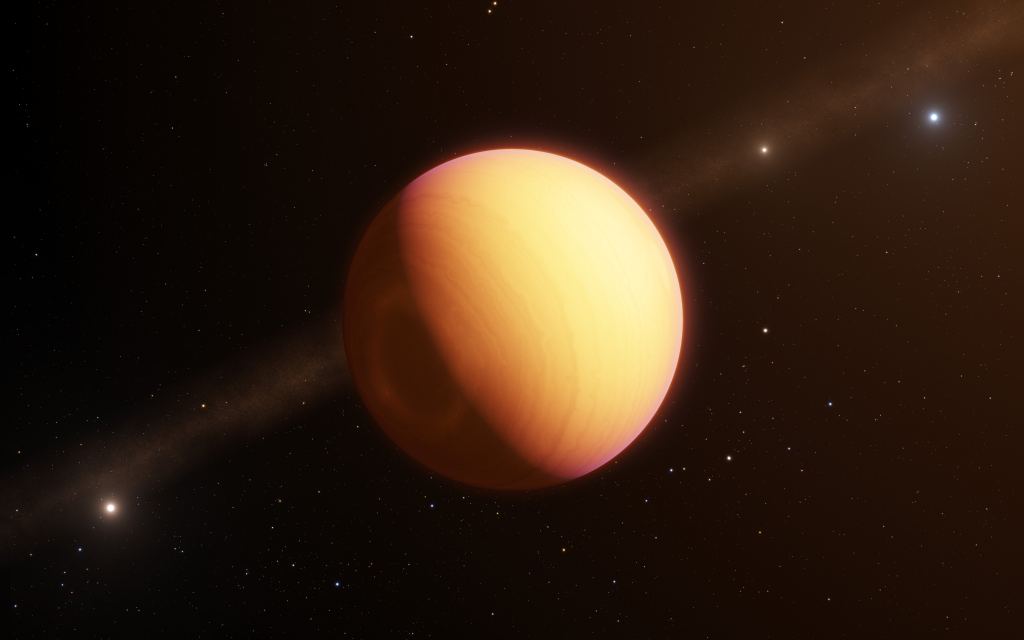
“Machine learning allows us to quickly identify optimal filters, as well as the trade-off in accuracy at various signal-to-noise ratios,” added Pham. “In the first task, using [the open-source algorithm] XGBoost, we get a ranking of which filters are most helpful for the algorithm in its tasks of detecting water, snow, or cloud. In the second task, we can observe how much better the algorithm performs with less noise. With that, we can draw a line where getting more signal would not correspond to much better accuracy.”
To make sure their algorithm was up to the task, Pham and Kaltenneger did some considerable calibrating. This consisted of creating 53,130 spectra profiles of a cold Earth with various surface components – including snow, water, and water clouds. They then simulated the spectra for this water in terms of atmosphere and surface reflectivity and assigned color profiles. As Pham explained:
“The atmosphere was modeled using Exo-Prime2 — Exo-Prime2 has been validated by comparison to Earth in various missions. The reflectivity of surfaces like snow and water are measured on Earth by USGS. We then create colors from these spectra. We train XGBoost on these colors to perform three separate goals: detecting the existence of water, the existence of clouds, and the existence of snow.”
This trained XGBoost showed that clouds and snow are easier to identify than water, which is expected since clouds and snow have a much higher albedo (greater reflectivity of sunlight) than water. They further identified five optimal filters that worked extremely well for the algorithm, all of which were 0.2 micrometers broad and in the visible light range. The final step was to perform a mock probability assessment to evaluate their planet model regarding liquid water, snow, and clouds from the set of five optimal filters they identified.

“Finally, we [performed] a brief Bayesian analysis using Markov-Chain Monte Carlo (MCMC) to do the same task on the five optimal filters, as a non-machine learning method to validate our finding,” said Pham. “Our findings there are similar: water is harder to detect, but identifying water, snow, and cloud through photometry is feasible.”
Similarly, they were surprised to see how well the trained XGBoost could identify water on the surface of rocky planets based on color alone. According to Kaltenegger, this is what filters really are: a means for separating light into discreet “bins.” “Imagine a bin for all red light (the “red” filter), then a bin for all the green light, from light to dark green (the “green” filter),” she said.
Their proposed method does not identify water in exoplanet atmospheres but on an exoplanet’s surface via photometry. In addition, it will not work with the Transit Method (aka. Transit Photometry), which is currently the most widely-used and effective means of exoplanet detection. This method consists of observing distant stars for periodic dips in luminosity attributed to exoplanets passing in front of the star (aka. transiting) relative to the observer.
On occasion, astronomers can obtain spectra from an exoplanet’s atmosphere as it makes a transit – a process known as “transit spectroscopy.” As the sun’s light passes through the exoplanet’s atmosphere relative to the observer, astronomers will analyze it with spectrometers to determine what chemicals are there. Using its sensitive optics and suite of spectrometers, the JWST will rely on this method to characterize exoplanet atmospheres.
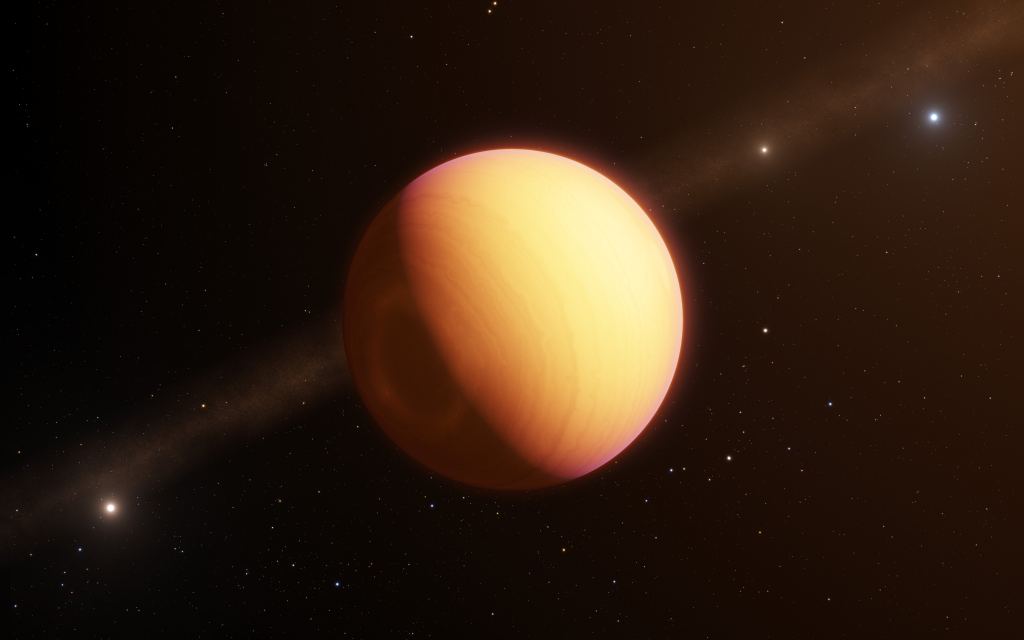
But as Pham and Kaltenneger indicate, their algorithm will only work with reflected light from the direct imaging of exoplanets. This is especially good news considering that spectroscopy obtained through Direct Imaging studies is likely to reveal more about exoplanets – not just the chemical composition of their atmospheres. According to Kaltenneger, this creates all kinds of opportunities for next-generation missions:
“This is opening up the opportunity for smaller space missions like the Nancy Roman telescope to help identify worlds that could host life. And for larger upcoming telescopes – as recommended by the decadal survey – it allows them to scan the rocky planets in the Habitable Zone for the most promising candidates – those with water on their surface, so we spend the time characterizing the most interesting ones – and effectively search for life on planets that have great conditions for it to get started.”
The paper that describes their findings was recently published in the Monthly Notices of the Royal Astronomical Society (MNRAS).
Further Reading: arXiv
The post Machine Learning Will be one of the Best Ways to Identify Habitable Exoplanets appeared first on Universe Today.

March 20, 2022 at 03:30AM
via Universe Today read more...
Post a Comment